3D object detection technology based on LiDAR is one of the most important pattern recognition tasks in the field of robot and autonomous driving. How to design robust feature expression according to the sparse and irregular point clouds, to improve the detection accuracy, is a key problem to be solved. Prof. Jianfeng Feng’s team at the Institute of Science and Technology for Brain-Inspired Intelligence, Fudan University, together with the Baidu vision technology team and Nanjing FAW autonomous driving team, proposed a general framework for 3D object detection based on the associative recognition mechanism of the human brain.
On August 11 (local time in the United States), a paper entitled “AGO-Net: Association-Guided 3D Point Cloud Object Detection Network”, was published online in the IEEE Transactions on Pattern Analysis and Machine Intelligence (TPAMI), the world's top journal in the field of artificial intelligence. This research work provides a new research idea for 3D object detection inautonomous driving.
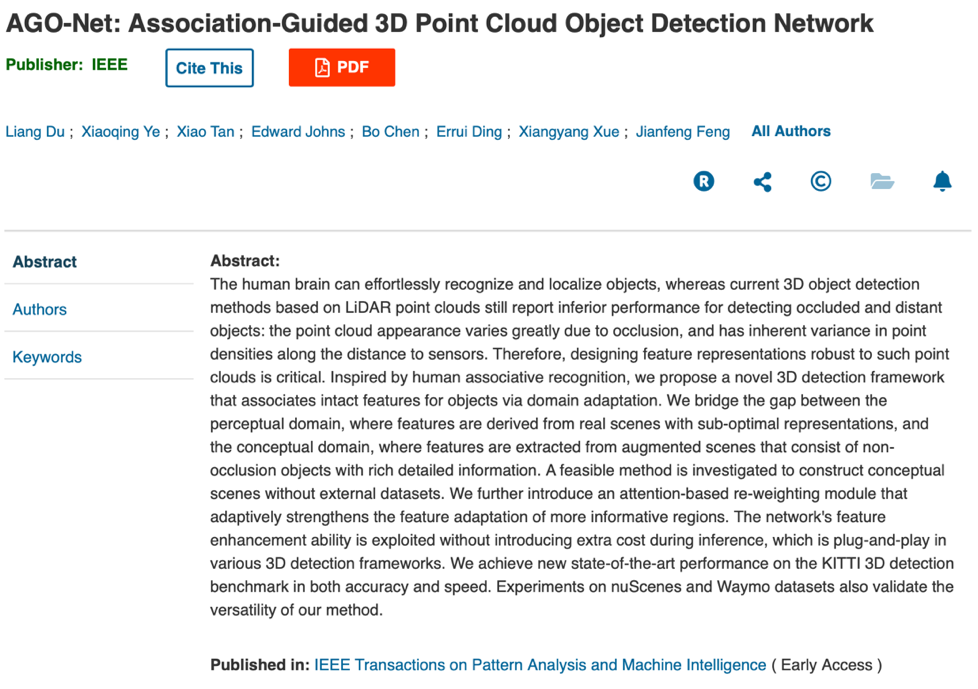
Figure 1. IEEE Explore (Early Access)
In the process of driving a vehicle, human beings can quickly and accurately judge the position and attitude of the vehicle no matter whether the vehicle in front is occluded or distant, which is due to the “Head Canon” ability of the brain. Only based on some approximate contour information and feature of the vehicle, the complete vehicle, and its position and attitude can be inferred and imagined according to the conceptual vehicle model stored in human memory. For the human psychological model, perceiving 3D objects is carried out in the form of a conceptual association process, which includes two vital stages:
(I) a “viewer-centered” feature representation stage, where the features of perceptual objects are presented from the viewer's perspective, and the visible features might lack structure and detail information due to occlusion and large distance, and (II) an “object-centered” stage, where the object's features are enhanced by the conceptual model of the same category retained in the memory. This association process helps map from an object to a view-invariant 3D conceptual model, which is termed as associative recognition.
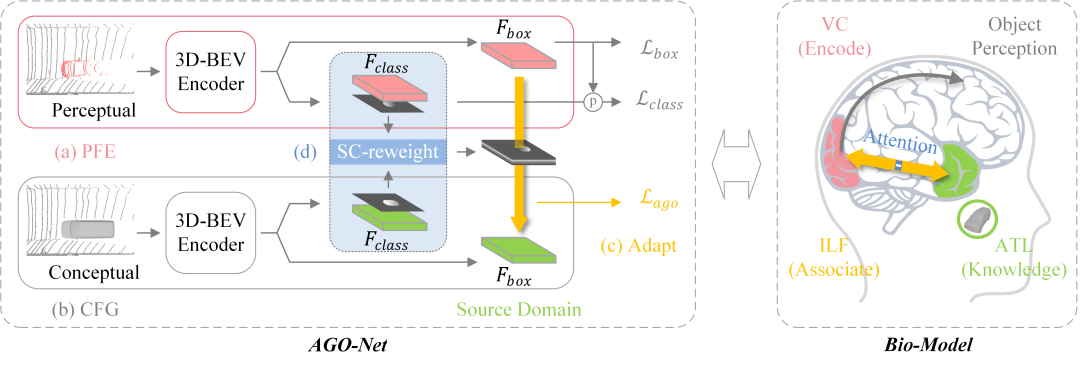
Figure 2.Overview Framework of AGO-Net
Figure 2 depicts the overall framework of AGO-Net, which includes four parts: (a) the perceptual feature encoder (PFE) to encode real-world target domain features for 3D object detection.
(b) the conceptual feature generator (CFG) to generate the source domain features from corresponding scenes reconstructed by the conceptual models.
(c) the perceptual-to-conceptual feature domain adaptation.
(d) the Spatial and Channel-wise loss reweighting module (SC-reweight) based on the classification features from both perceptual and conceptual scenes, which forces the network to strengthen the adaptation for regions that have much more critical information.
To sum up, AGO-Net bridges the gap between the perceptual domain, where features are derived from real scenes with sub-optimal representations, and the conceptual domain, where features are extracted from augmented scenes that consist of non-occlusion objects with rich detailed information, which simulates the associative recognition of the human brain.The network's feature enhancement ability for sparse and occluded point clouds is exploited without introducing extra cost during inference, which can be easily integrated into various 3D detection frameworks.
The proposed method achieved new state-of-the-art performance on the KITTI 3D detection benchmark in both accuracy and speed. Experiments on nuScenes and Waymo datasets also validate the versatility of the method.
The first author of the present study is Liang DU, a Ph.D. candidate from the Institute of Science and Technology for Brain-Inspired Intelligence (ISTBI) at Fudan University, and the corresponding author is Prof. Jianfeng Feng. The relevant work has been supported by the municipal science and technology major project of "Basic Transformation and Application Research of Brain and Brain-inspired Intelligence" in Shanghai, the National Natural Science Foundation of China, and the Key Laboratory of Computational Neuroscience and Brain-inspired Intelligence of the Ministry of education.
Article link:https://ieeexplore.ieee.org/document/9511841