Recently, research teams led by Dr. Xiao-Yong Zhang at ISTBI and Dr. Tingying Peng at Helmholtz AI developed an deep learning-based framework, MouseGAN++, for simultaneous image synthesis and segmentation for mouse brain MRI. Based on a disentangled representation of content and style attributes strengthened by contrastive learning, MouseGAN++ is able to synthesize multiple MR modalities from single ones in a structure-preserving manner and can hence handle cases with missing modalities. Furthermore, it uses the learned modality-invariant information to improve structural segmentation. The study was published in IEEE Transactions on Medical Imaging on November 30, 2022.
The multi-modality structure images of the mouse brain were acquired with a 11.7T MR scanner at Zhangjiang Imaging BrainBank, Fudan University, including T2w imaging, T1w imaging, T2*w imaging, quantitative susceptibility mapping (QSM), and Magnitude MR images (Mag). This dataset covered 75 mouse brains with 56,970 MR slices.
A novel synthesis-and-segmentation model, MouseGAN++, comprising modality translation module based on feature disentanglement and contrastive learning to synthesize all modalities, and semantic segmentation module to get fine brain structures.
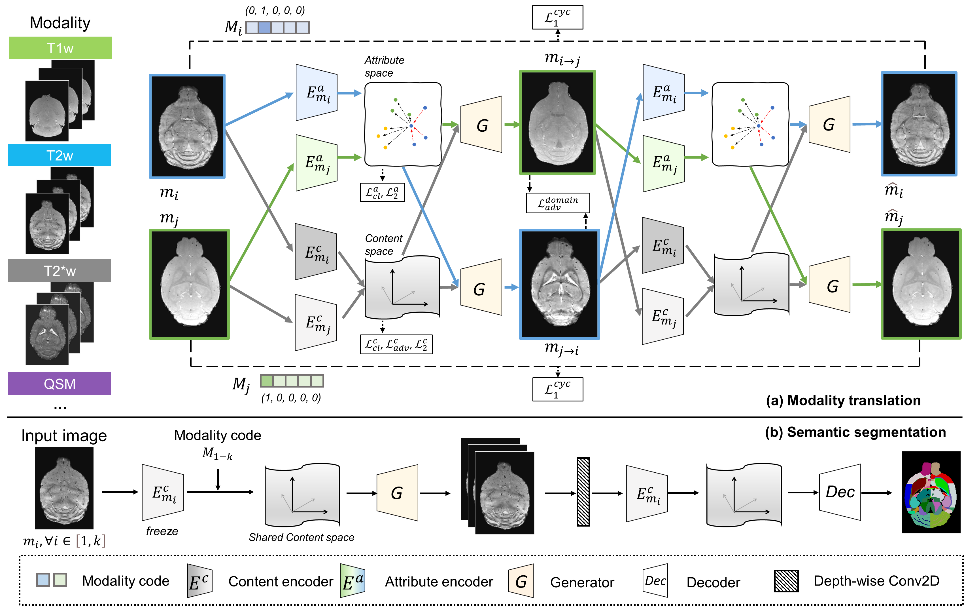
△Schematic of MouseGAN++.
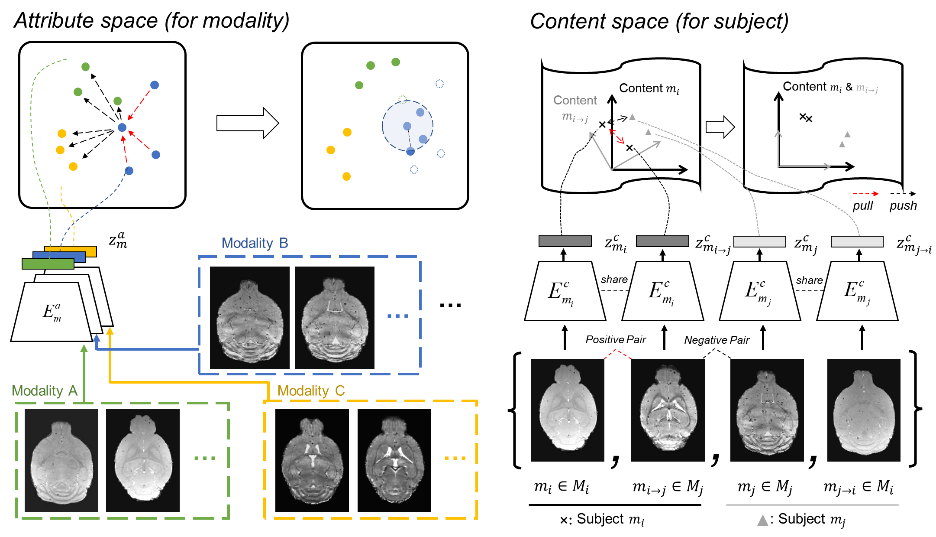
△Illustration of the attribute discrimination (left) and content discrimination (right) achieved by contrastive learning.
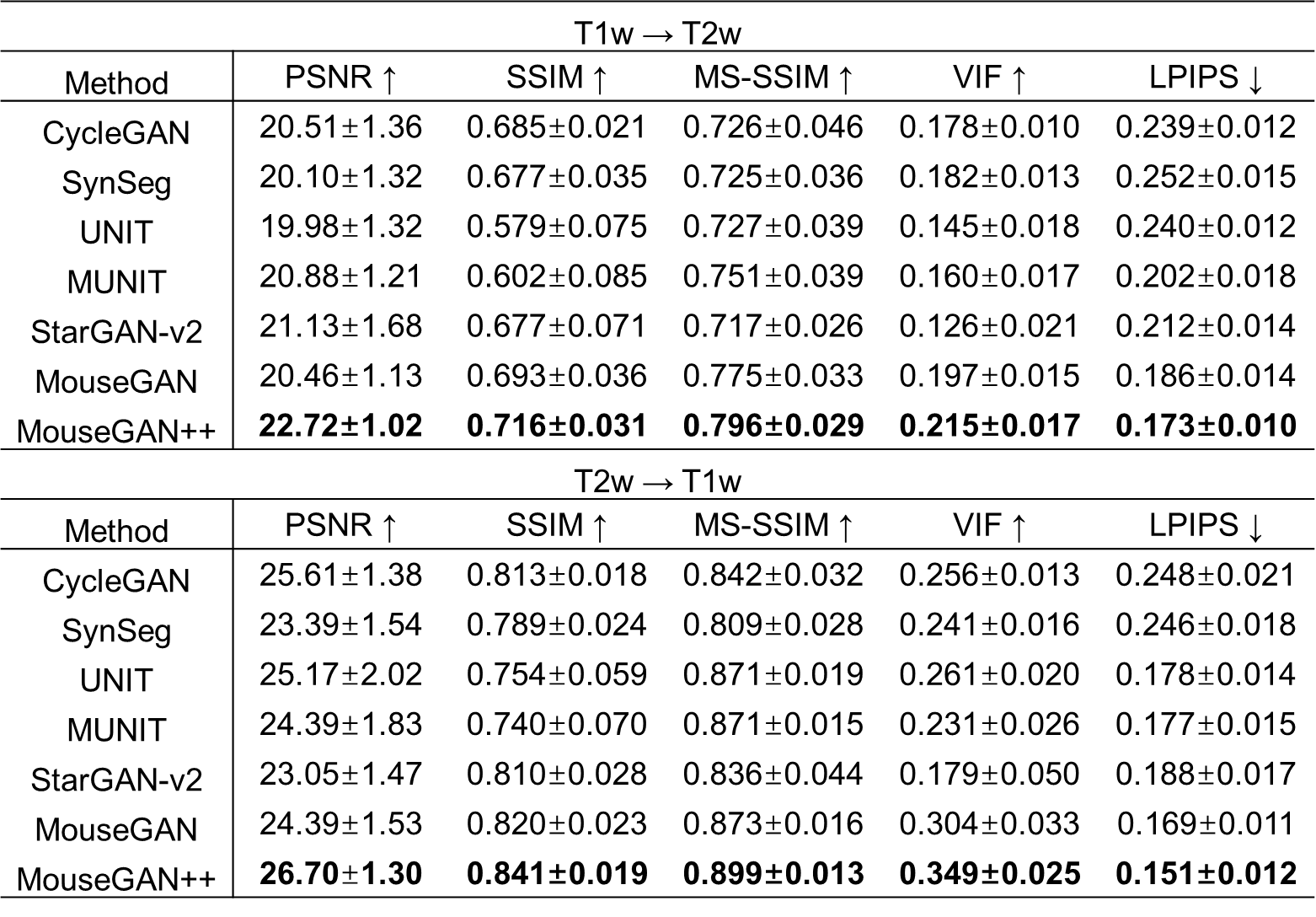
△Translation performance comparison with different methods from T1w to T2w and vice versa
In the above Table, for two-direction translations, MouseGAN++ surpasses other methods in terms of all five metrics that are used to measure image synthesis quality. More specifically, for the translation from T1w to T2w, MouseGAN++ achieves the best performance with the highest SSIM of 0.716 and the lowest LPIPS of 0.173. Besides, MouseGAN++ produces sharper and more realistic textures, with the content better aligned with anatomy than other methods.
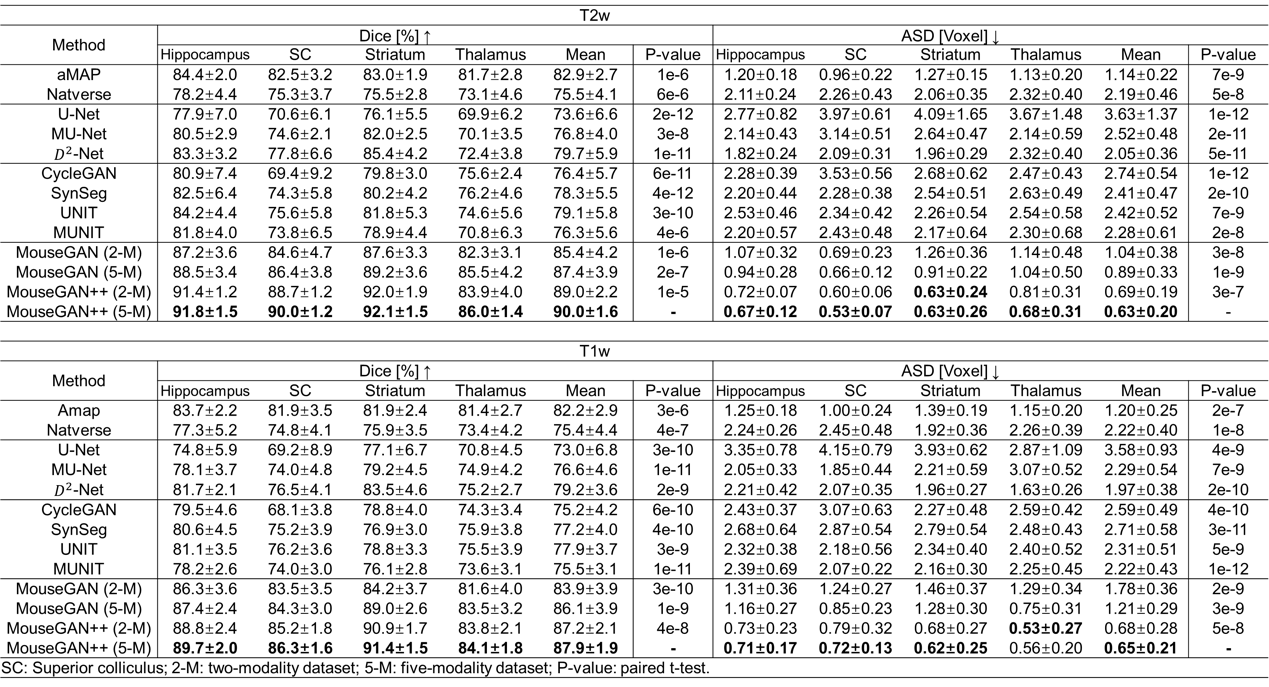
△Comparison of segmentation performance of different methods on T1w and T2w using multi-modality dataset.
The quantitative results of brain structural segmentation on T1w and T2w, the two most important MR modalities used in mouse brain studies, are summarized in the following Table. In both modalities, MouseGAN++ consistently surpasses comparison methods, demonstrating the effectiveness of our proposed framework.
Our results demonstrate that MouseGAN++ achieves significant performance improvement over both translation and segmentation tasks and has the potential to be applied in more neuroimaging applications. We release our method as a packaged tool for free academic usage at https://github.com/yu02019.
Full Article:
Z. Yu, X. Han, S. Zhang, J. Feng, T. Peng and X. -Y. Zhang, "MouseGAN++: Unsupervised Disentanglement and Contrastive Representation for Multiple MRI Modalities Synthesis and Structural Segmentation of Mouse Brain," in IEEE Transactions on Medical Imaging, doi: 10.1109/TMI.2022.3225528.