Recently, Prof. Junping Zhang at the School of Computer Science and Prof. Jianfeng Feng at the Institute of Science and Technology for Brain-Inspired Intelligence, Fudan University, established a joint research team to propose a GaitSet theory and corresponding algorithm. The method provides a new perspective for employing gait recognition on the natural world and achieves breakthroughs in biometrics.
With the popularity of HD cameras and the maturity of artificial intelligence, biometricsauthentication, especially face recognition, has become an essential method to identify persons in airports, high-speed railways, mobile payments, and other applications. However, face recognition is vulnerable to be attacked despite its high recognition rate, and heavily suffers from illumination and posture variants. Further, face recognition cannot function when the distance is more than 7 to 8 meters. As for other biometrics, fingerprints commonly used in access control systems is not touchy-free. Meanwhile, Iris can be recognized at a distance of no more than 1.5 meters and is vulnerable to contact lens products. These problems, plagued by the most commonly used biometric authentication technology, resulted in significant recognition performance degradation. Therefore, there is always a need to propose biometric authentication systems which are more resistant to attack at long distance and substantial changes in illumination circumstances.
Gait, the posture of a person walking, is composed of periodic striding, stepping, and closing movements. Psychological studies have shown that each person's walking posture is different and thus can be used for identification. In addition, gait can effectively identify people from 20 meters away and is the unique biometric feature that can be identified from a distance. The recognition mechanism is similar to human fast-thinking cognition that one only needs to see the silhouette to quickly identify a familiar person without having to recall many details about him or her too carefully. For example, video gait recognition helped to arrest the criminal Zhou Kehua in the famous Su Xiangyu series of armed robbery and murder cases. Additionally, gait is the natural walking posture of a person in an unsuspecting situation and is not easy to hide. However, gait recognition is easily influenced by road conditions such as concrete and lawn and external factors such as whether one is wearing a backpack or a different dressing. Therefore, relevant research and practical applications need to be further advanced.
There are two commonly biometric authentication strategies for gait recognition. One is based on the video sequence formed by the periodicity of gait walking that the gait is recognized by comparing frame by frame. This method has high performance but low recognition efficiency. The other is to average the video gait sequences to form a gait energy map template and recognize them based on this template. This method has real-time efficiency, but the recognition performance is not as good as the aforementioned method. Besides, the gait captured by the camera is often from multiple views in a real-world environment. Therefore, it will be difficult to extend to practical applications if the algorithm can only be at a single view.
Since the Ministry of Science and Technology awarded Prof. Junping Zhang the Exploratory Project in 2006, his team has accumulated nearly 16 years of research experiences on gait recognition and published high-quality papers in top pattern recognition journals, such as IEEE TPAMI, IEEE TSMCB, and ECCV. He obtained a series of research results in low-resolution gait recognition and temporal consistency templates. The temporal consistency template, i.e., CGI (Chrono Gait Image) was tested in 2012 for a third party in the gait common dataset OU-ISIR, which was listed as one of the six state-of-the-art algorithms and got the third ranking.
Based on the above results, Prof. Junping Zhang and Prof. Jianfeng Feng, the director of the Brain-like Research Institute, formed a joint research team to further think beyond the previous gait recognition to obtain the best performance and potential utility. They discard the traditional gait cycle approach and view the gait sequence as a set, subverting the GaitSet theory and algorithm. As a result, the method is insensitive to the frame order within one sequence and can achieve maximum recognition performance with a few gait image inputs.
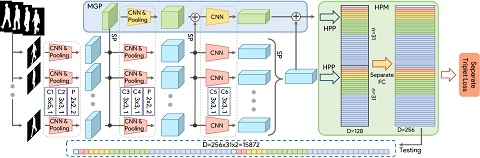
According to the researchers, GaitSet neither compresses the gait sequence into a single image nor views it as a standard video sequence. Instead, the gait sequence is regraded as a collection composed of silhouettes. This improvement gives a massive advantage to the model, making it significantly outperform all existing algorithms. For example, most previous methods do not exceed 70% accuracy when using 62 individuals for training on the CASIA-B, a standard dataset for gait recognition. GaitSet achieves close to 80% accuracy using only 24 individuals, over 90% using 62 individuals. Using 74 individuals for training reaches 95% accuracy, 3% higher than the known single model best result in the field of gait recognition. The high performance of GaitSet achieves the practical application metric performance demand. GaitSet also achieves optimal performance on the other two standard datasets. What's more, GaitSet introduces some new properties to the model. On the one hand, by relaxing the restriction of sequences to sets, frames collected from different places can be put together as input. As a result, the model can fuse the information in different structures to obtain higher accuracy. On the other hand, the model can handle arbitrary-sized sets, from as little as one frame up to a maximum. Furthermore, recognition the accuracy increases gradually as the number of frames increases. It is worth noting that these new features are natural to the model and do not require targeted training.
The work was published at AAAI 2019, the top conference on artificial intelligence, and orally presented on February 2, 2019. And an extended version was also published in IEEE TPAMI, 2012.
GaitSet will be a landmark in gait recognition, opening new perspectives for gait recognition to become more practical. Furthermore, this recognition will also contribute to the study of the formation of human cognitive models such as large-scale prioritized mental concepts.