Face recognition has been a hot research topic in computer vision for many years. Despite the remarkable success of general face recognition (GFR), how to minimize the impact of age variation is a lingering challenge for current face recognition systems in correctly identifying faces in many practical applications such as tracing long-missing children. Therefore, it is of great significance to achieve face recognition without age variation, i.e., age-invariant face recognition or AIFR. AIFR, however, remains extremely challenging in the following three aspects. First, when the age gap becomes large in cross-age face recognition, age variation can dominate the facial appearance, which then significantly compromises the face recognition performance. Second, face age synthesis (FAS) is a complex process involving face aging/rejuvenation (a.k.a age progression/regression) since the facial appearance changes dramatically over a long time and differs from person to person. Last, it is infeasible to obtain a large-scale paired face dataset for training a model to render faces with natural effects while preserving identities.
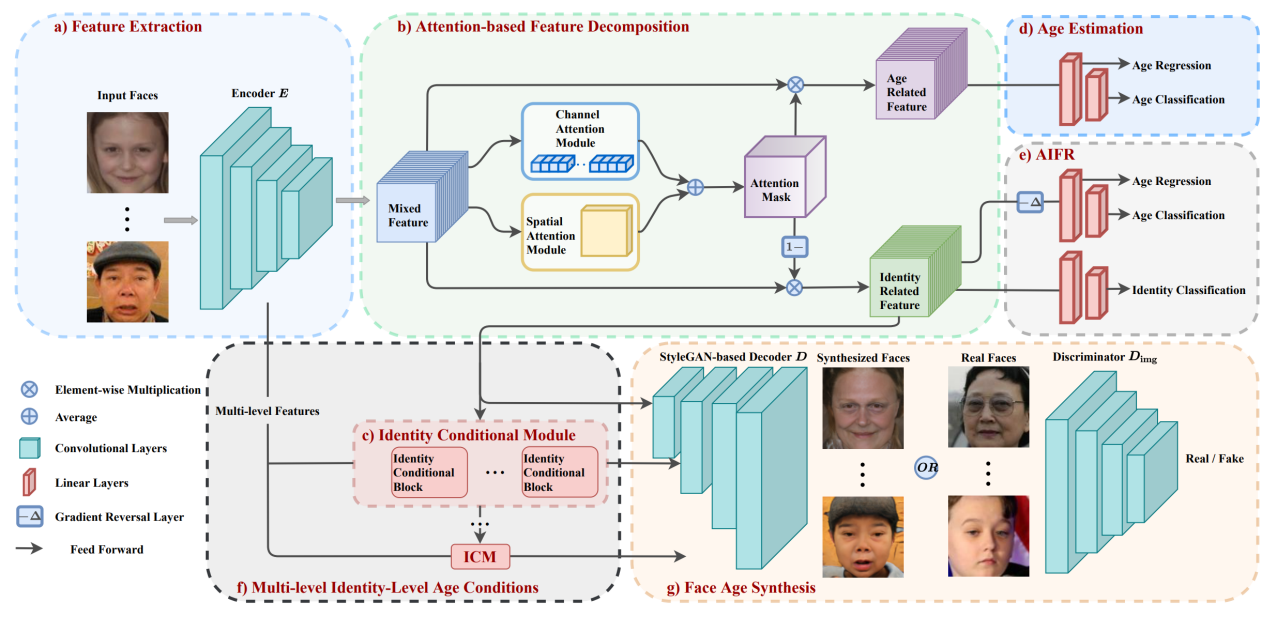
Fig. 1 An overview of the proposed MTLFace
In a paper published in IEEE Transactions on Pattern Analysis and Machine Intelligence, Dr. Hongming Shan at ISTBI and Prof. Junping Zhang at School of Computer Science propose a unified, multi-task learning framework to simultaneously achieve AIFR and FAS, termed MTLFace, which can enjoy the best of both worlds; i.e., learning age-invariant identity-related representations while achieving pleasing face synthesis. More specifically, the researchers propose an attention-based feature decomposition to decompose the mixed high-level features into two uncorrelated components—identity- and age-related features—in a spatially constrained way. they then decorrelate these two components in a multi-task learning framework, in which an age estimation task is to extract age-related features while a face recognition task is to extract identity-related features; in addition, a continuous cross-age discriminator with a gradient reversal layer further encourages extracting identity-related age-invariant features. Moreover, they propose an identity conditional module to achieve identity-level transformation patterns for FAS, with a weight-sharing strategy to improve the age smoothness of the synthesized faces; i.e., the faces are aged smoothly. Extensive experimental results demonstrate the superior performance of the proposed MTLFace over existing state-of-the-art methods for AIFR and FAS, and competitive performance for general face recognition in the wild.
Deep clustering aims to learn the representation of images and perform clustering in an end-to-end fashion. Existing deep clustering methods heavily rely on contrastive representation learning. Although achieving promising results, contrastive-based methods usually require a large number of negative examples to learn uniform representations. The involved negative pairs may inevitably lead to the class collision issue. An alternative perspective is to separate the typical contrastive loss into two terms: 1) alignment term to improve the closeness of positive pairs, and 2) uniformity term to encourage instances to be uniformly distributed on a unit hypersphere by pushing away the negative pairs. Apparently, the uniformity term could introduce class collision issue. Different from contrastive learning, non-contrastive learning only involves the alignment term using the representations of one augmented view to predict another. The non-contrastive learning can avoid the class collision issue as there are no negative pairs. Lacking the uniformity term in contrastive loss, it is not guaranteed to learn uniform representations.
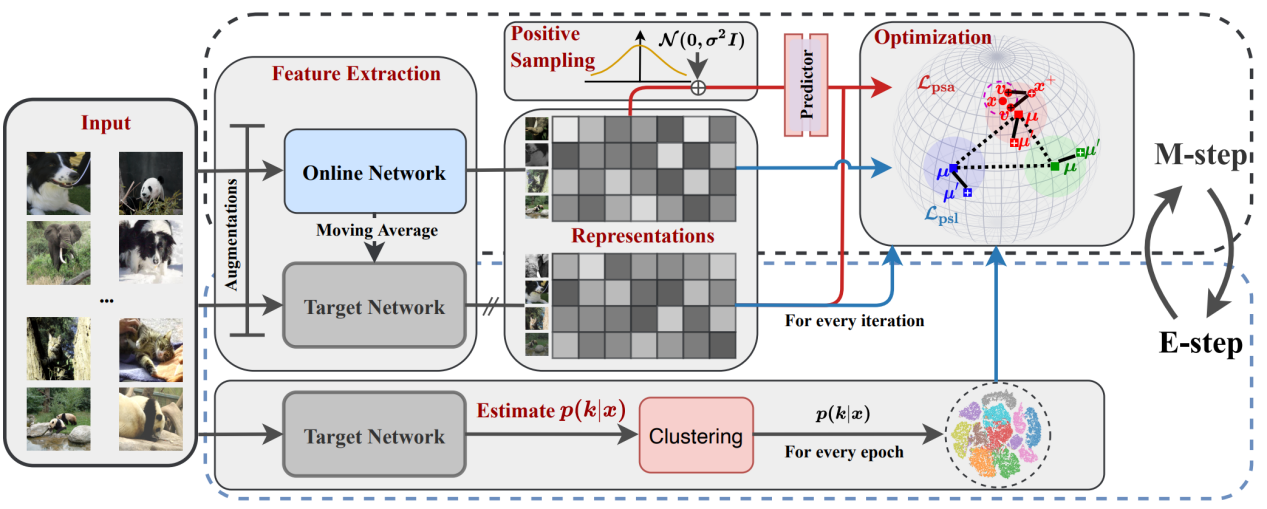
Fig. 2 The overall framework of the proposed ProPos in an EM framework
In this work, the researchers propose a novel end-to-end deep clustering method, ProPos, with two novel techniques: prototype scattering loss and positive sampling alignment. First, we propose to perform contrastive learning over prototypical representations, in which two augmented views of the same prototypes are positive pairs and different prototypes are negative pairs. This yields the proposed prototype scattering loss or PSL, which maximizes the between-cluster distance so as to learn uniform representations towards well-separated clusters. Second, they further propose to align one augmented view of the instance with the randomly sampled neighbors of another view that are assumed to be truly positive pairs in the embedding space, which they refer to as positive sampling alignment or PSA. Compared to conventional alignment between two augmented views, the proposed PSA takes into account the neighboring samples in the embedding space, improving the within-cluster compactness. Extensive experimental results on several benchmark datasets demonstrate that ProPos outperforms the existing state-of-the-art methods by a significant margin, especially for large-scale datasets.
Full Article:
Z. Huang, J. Chen, J. Zhang and H. Shan, "Learning Representation for Clustering Via Prototype Scattering and Positive Sampling," in IEEE Transactions on Pattern Analysis and Machine Intelligence, 2022, doi: 10.1109/TPAMI.2022.3216454.