Signal propagation in complex networks plays a vital role in driving epidemics, promoting trust and moral behavior in social groups, enabling the development of misinformation detection algorithms, and supporting the cognitive abilities of the brain. The geometry of signal propagation is influenced by both network topology and diverse forms of nonlinear interactions between nodes. However, advances in this area are often system-dependent and have limited translational potential across domains. Given over two decades of research on this subject, there is an urgent need for a comprehensive review.
A research team, led by Prof. Peng Ji from the Institute of Science and Technology for Brain-Inspired Intelligence of Fudan University, reviewed the research progress of signal propagation in complex networks and its application in various fields in Physics Reports on 4 April 2023.
Signal propagation in complex systems can be taken as the continuous transmission of information, physical quantities, or even states between units. The dynamical model is the basic mathematical tool that specifically describes this interaction mechanism, and a concise but accurate dynamics model is the first step in understanding signal propagation. The authors introduce the construction methods and research progress of various common theoretical models that carry signal propagation phenomena, including epidemic models, Kuramoto models, diffusion models, cascading failure models, and models describing neuronal dynamics.
On the other hand, the underlying topologies determine many local properties of signal propagation such as direction and speed. The authors point out that, compared to traditional random networks, emerging network structures, such as time-varying and multilayer networks, have a more complex impact on the topological factors caused by signal propagation, but they also provide new ideas and methods for network regulation and intervention in the propagation process. Based on a general kinetic model, the corresponding theoretical framework successfully separates the factors of kinetics and topology, which is of great significance for deepening the understanding of the roles played by both in signal propagation.
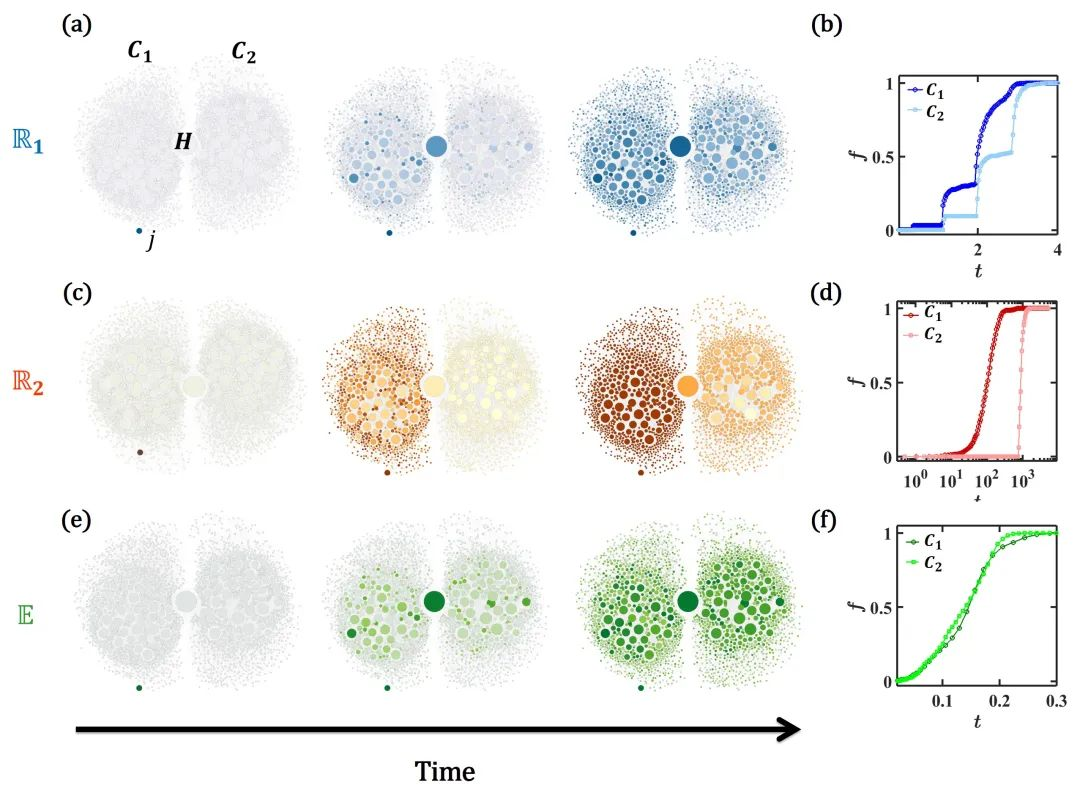
Fig.1 Different spreading patterns is constructed with different dynamical models
In contrast to traditional research that starts from theoretical systems composed of models and networks, big data and the rapid development of data analysis techniques have made it possible to reverse-analyze signal propagation from massive amounts of data. The critical issue is how to correctly restore the connection between units under an unknown system structure from time series data and then identify the path of signal propagation. From the most basic linear correlation to non-linear correlation, and then to the introduction of information flow and transfer entropy concepts, many time series analysis techniques have laid a solid foundation for analyzing signal propagation.
After reviewing a series of theoretical and numerical methods for analyzing unit dependency, the authors further introduce the latest progress in signal propagation analysis, including structure and functional network reconstruction represented by causal inference, information source localization based on time-varying network systems, unit connection inference, and signal processing and time series prediction methods driven by artificial intelligence. In the future, with the further enrichment of data, these analysis techniques will inevitably become important tools for deep analysis of signal propagation and promote a deeper understanding of various complex systems.
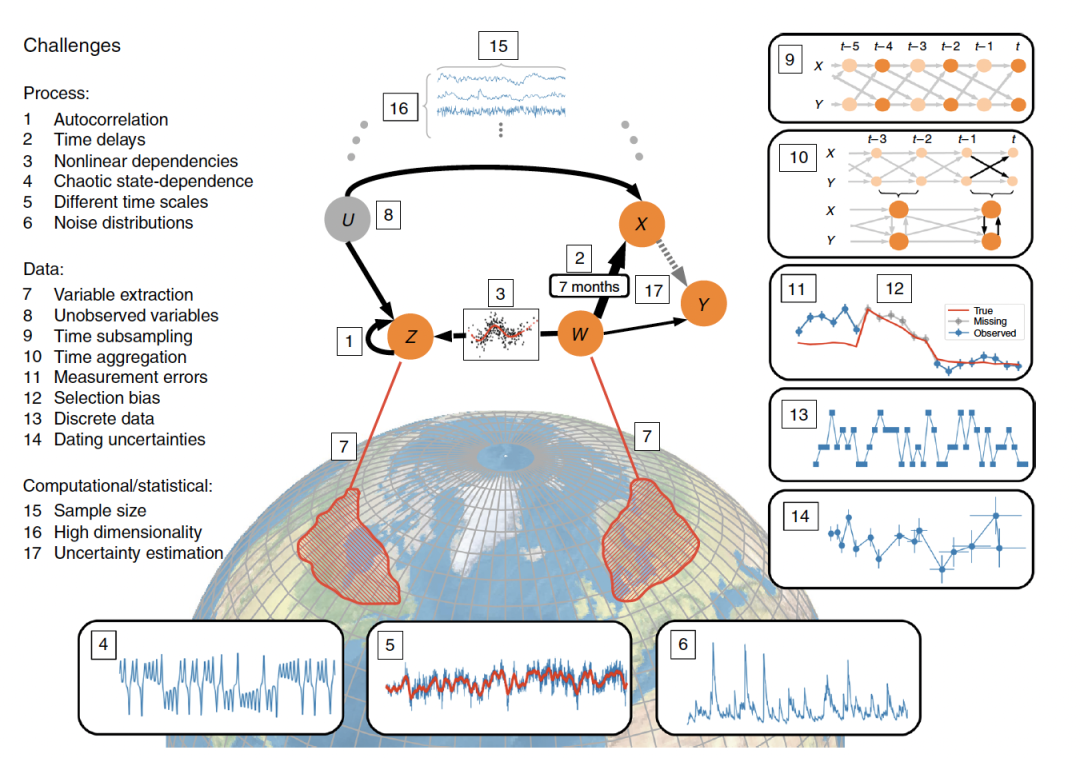
Fig. 2 Opportunities and challenges of causal network modeling of the global climate
Finally, the authors review the application of the aforementioned theories in fields such as epidemiology, social dynamics, neuroscience, power systems, and robotics, indicating that signal propagation research has broad application prospects. Taking neuroscience as an example, a large number of neural circuits constitute the most basic signal processing and transmission units in the brain. Under the unified integration of hierarchical networks, signals are transmitted in a certain direction to complete the execution of a brain task. The establishment of neural kinetic models and the collection and analysis of neural firing data help researchers understand neural signal transmission from different perspectives, which is conducive to a deep understanding of the brain and provides a theoretical basis for treating cognitive disorders and other neurological diseases.
This review not only helps to further promote the development of complex systems research but also provides a guide for future research.
Full Article:
Ji, P., Ye, J., Mu, Y. et al. Signal propagation in complex networks. Phy Rep 1017, 1–96 (2023). https://doi.org/10.1016/j.physrep.2023.03.005
About Our Author:
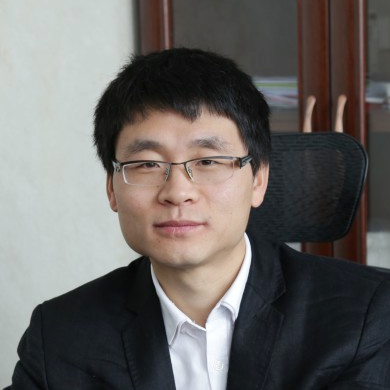
Prof. Peng Ji (First Author)
Professor
Prof. Peng Ji received his Ph.D. in theoretical physics from Humboldt University of Berlin in 2015 with Professor Jurgen Kurths, and then worked at the Potsdam Institute for Climate Impact Research in Germany. He joined ISTBI at the end of 2016 as Young Principal Investigator and became Professor in 2021. He has won many honorary titles such as Pujiang Talent (2017), Shanghai University Distinguished Professor-Oriental Scholar (2018), Oriental Scholar-Tracking Program (2021). His current research interests include human brain and zebrafish imaging analysis, neural network models, nonlinear dynamics analysis of complex networks, etc. He has published a series of articles in journals such as Nature Physics, Nature Communications, Physics Reports, Physical Review Letters as the first or corresponding author in recent years.